From gaming to cutting-edge biology: AI and the protein folding problem Understand article
How can AI systems like those developed to beat humans at games help unlock the secrets of protein function?
Proteins fold into complex 3D structures. Determining these structures is key to understanding many biological processes, but it requires time-consuming and expensive experiments. Scientists have been trying to computationally predict protein folds for about 50 years but progress has been slow and success limited. After developing game-playing artificial intelligence (AI) systems that beat humans in the abstract board game Go or Blizzard’s Starcraft, the Google-owned company DeepMind recently developed AlphaFold2, an AI system that is able to predict lots of protein structures with experimental accuracy. The following article explains the importance of this exciting breakthrough.
What do proteins look like?
Proteins are key to almost all biological processes. There are roughly 20 000 different proteins in the human body and millions on earth, and each one has a unique structure.
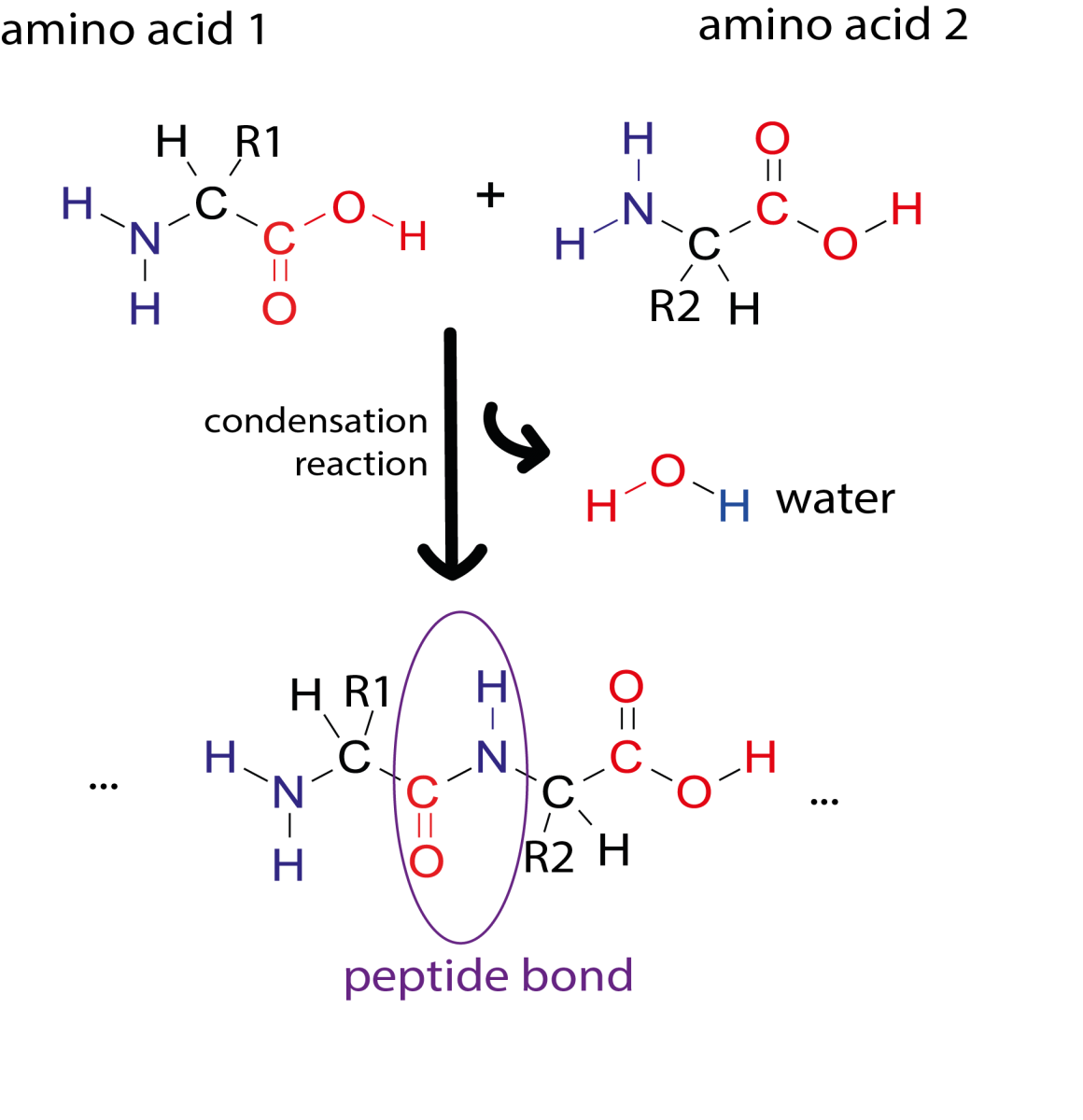
Image courtesy of Simone Heber
The primary structure of a protein consists of a sequence of amino acids connected by peptide bonds. Proteins are built from 20 different amino acids and their sequence is encoded in our DNA. Sections of an amino acid chain can fold into secondary structures, like α-helices and β-sheets. These secondary structures can then interact with each other to form complex 3D shapes (tertiary structure). Due to the way the protein chain folds around itself, amino acids that are far apart in the primary sequence may be close together in the 3D structure.
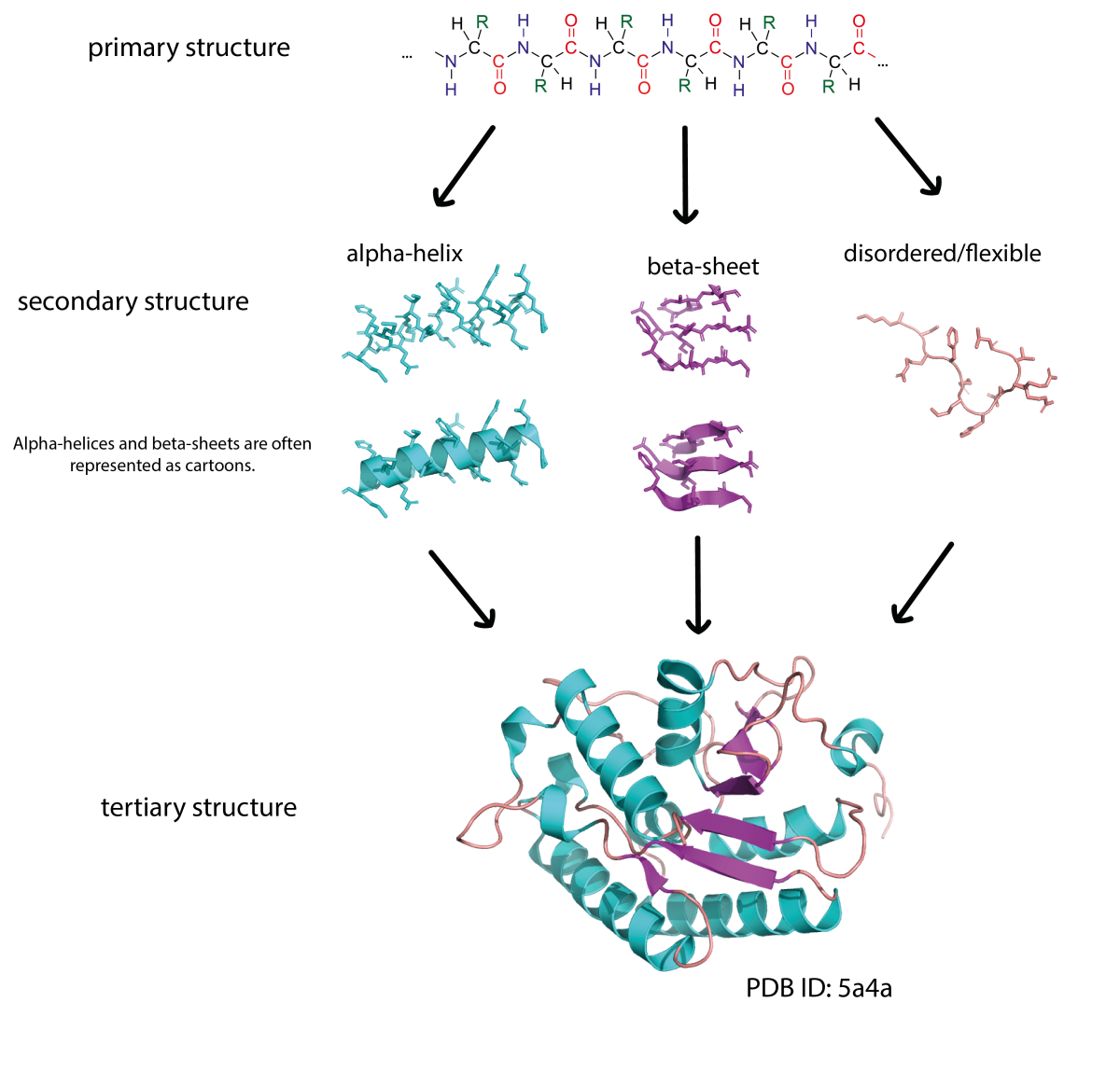
Image courtesy of Simone Heber
The 3D structure of a protein determines its function, and if folding goes wrong, it can lead to malfunction and disease. Diseases like Alzheimer’s and Parkinson’s disease are connected to misfolded proteins.
Solving the 3D structures of proteins is key to understanding the fundamental functions of life and can help to tackle disease. For example, the structure of a disease-linked protein can guide the design of an effective drug.
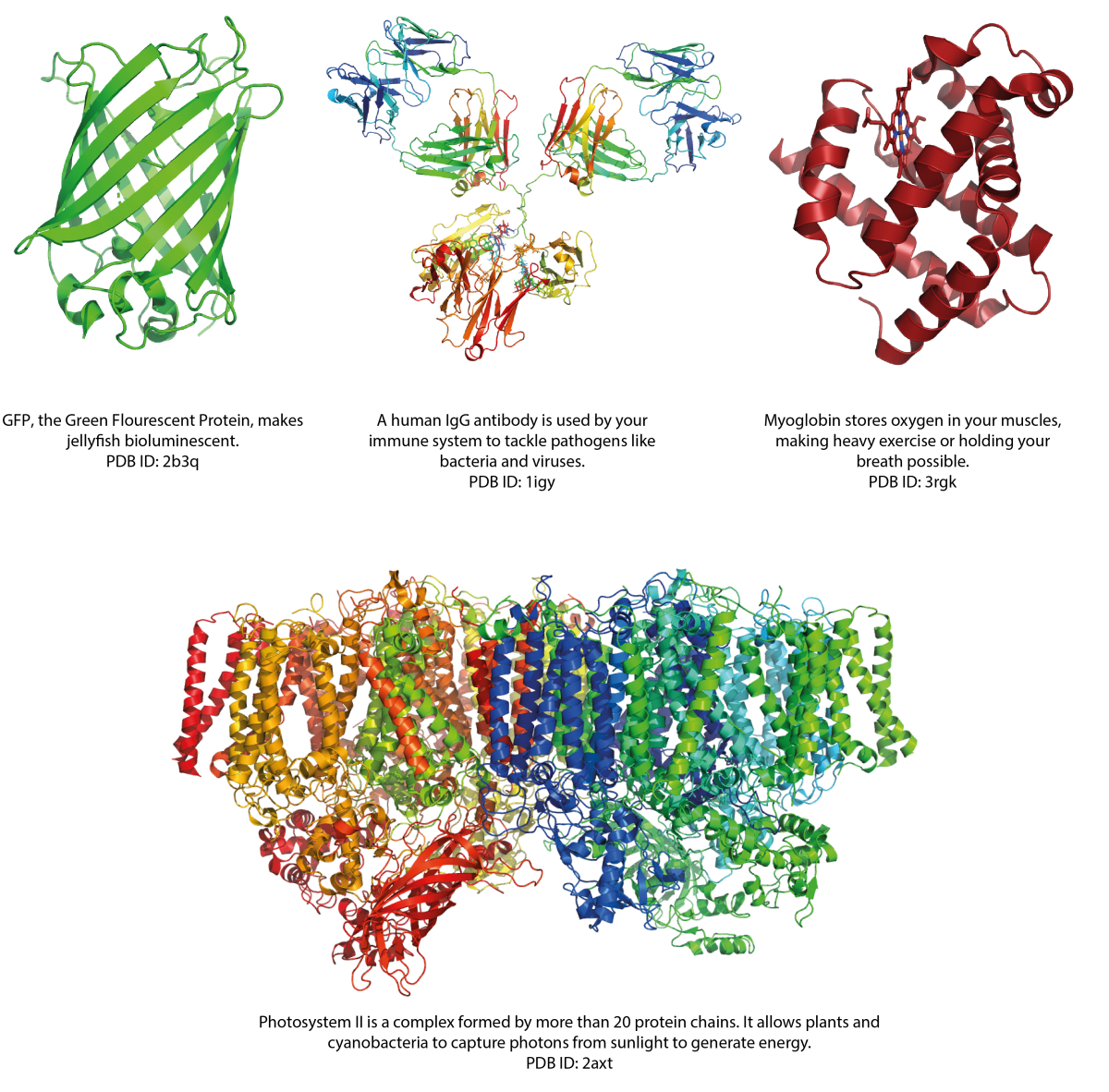
Image courtesy of Simone Heber
Experimental methods to determine protein structures
In 1962, Max Perutz and John Kendrew (who later became one of EMBL’s founders and its first director) received the Nobel Prize in Chemistry for determining the first protein structure, that of myoglobin, by X-ray crystallography.[1]
X-ray crystallography relies on shooting protein crystals with very bright X-rays, for example from synchrotron light sources, although some universities also have smaller, less powerful instruments. The protein crystal diffracts the X-rays and the diffraction pattern allows scientists to calculate the protein’s structure.
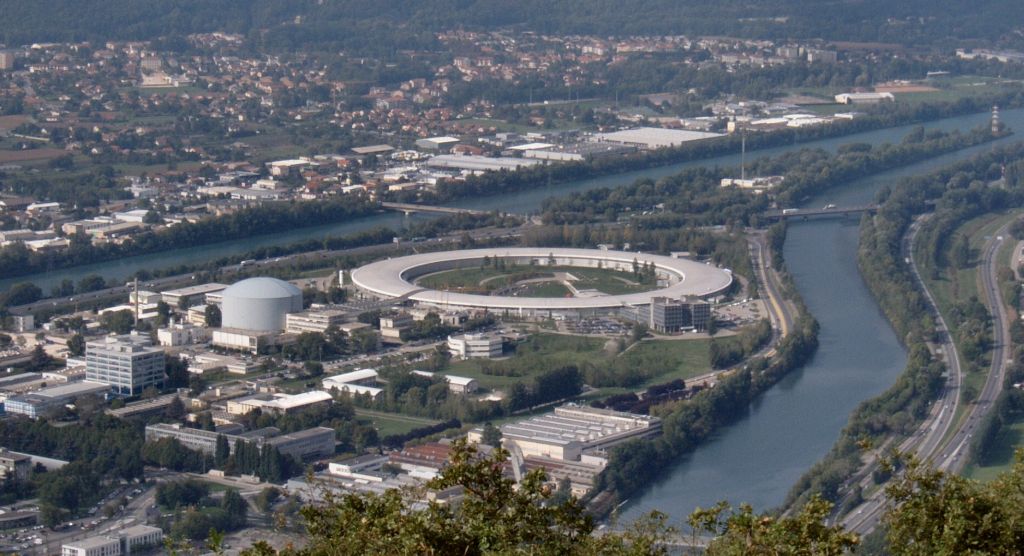
Image: Christian Hendrich, GNU Free Documentation License, Version 1.2
Image courtesy of Simone Heber
Image courtesy of Simone Heber
A second method is nuclear magnetic resonance (NMR) spectroscopy, which measures the magnetic fields of atomic nuclei. These magnetic fields are influenced by the atoms’ surroundings, so for atoms in proteins, they reveal information about which amino acids are close to each other. The 2002 Nobel Prize in Chemistry was awarded for this work.[2]
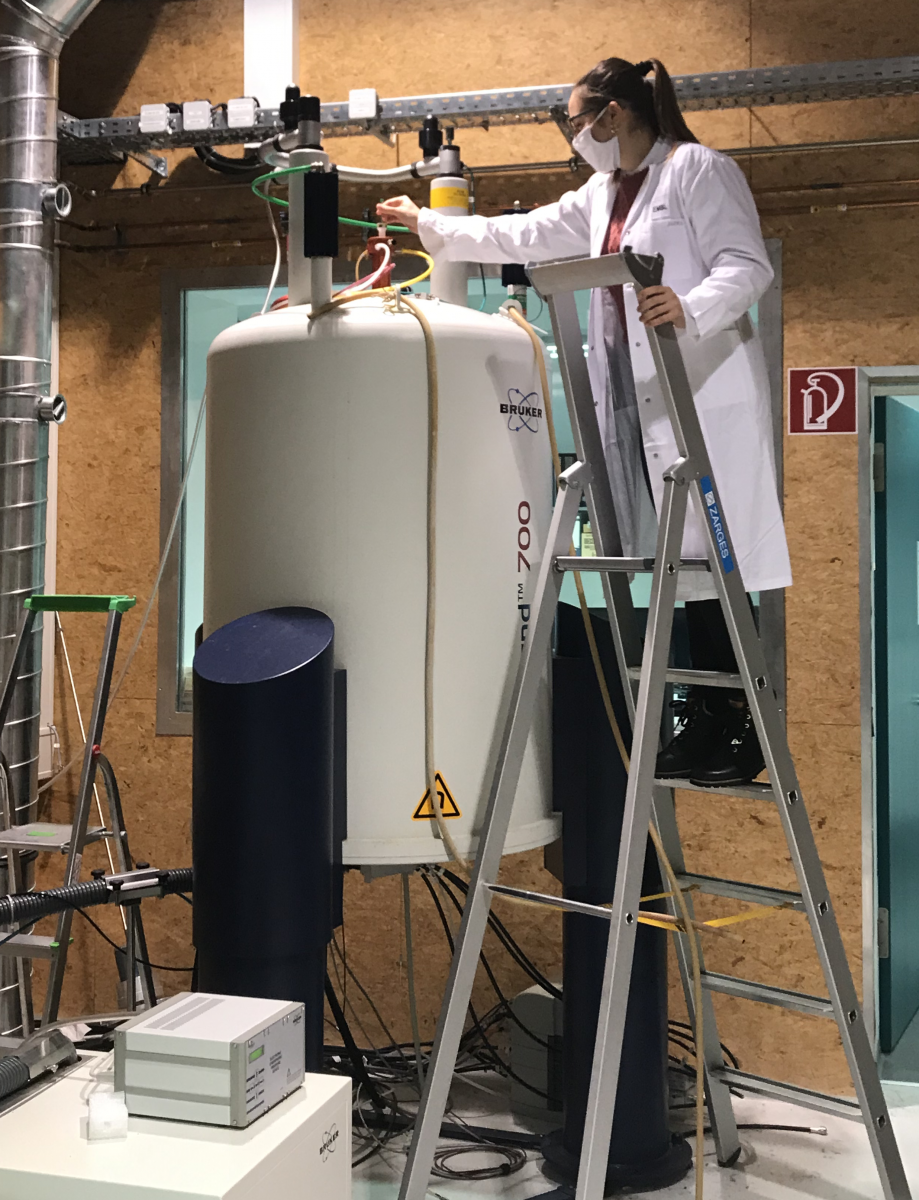
Image courtesy of Simone Heber
A third powerful method is cryogenic electron microscopy (cryo-EM), which is carried out on flash-frozen samples, for which the 2017 Nobel Prize in Chemistry was awarded.[3] In electron microscopy, a beam of electrons is used instead of light, which allows smaller details to be resolved.
These methods have allowed scientists to determine over 150 000 protein structures and make them publicly available in the Protein Data Bank (PDB).[4] However, these techniques are slow, expensive, and often limited by the nature of the protein. Scientists can spend years trying to solve a protein structure without any guarantee of success.
Why can’t we calculate a protein’s 3D structure from the primary structure?
In 1972, Christian B. Anfinsen received the Nobel Prize in Chemistry for showing that a protein’s sequence determines its structure.[5] The idea of predicting the 3D structure of a protein from its amino acid sequence has been around for about 50 years, and with the human DNA sequence known, primary structures of proteins are widely available. So why has there been little success so far?
A chain of amino acids can theoretically fold into an enormous number of tertiary structures: for a protein with 100 amino acids, there are an estimated 10300 (that’s a 10 with 299 zeros!) possible structures. In nature, proteins generally fold into the most stable structure. This minimum-energy structure can be calculated, however, comparing all possible structures requires an enormous amount of computing power. Efforts to solve the “folding problem” include the distributed computing project Folding@home,[6] which has become one of the world’s fastest computing systems by borrowing computing power from volunteers. You can get involved and help scientists by contributing unused CPU on your computer, smartphone, or PlayStation3 to protein folding!
The CASP competition and its 2020 winner AlphaFold
The Critical Assessment of protein Structure Prediction (CASP) forum was formed 1994[7] and holds a biannual competition in which scientists use software to predict protein structures that have been experimentally solved but not yet published. However, none have so far been able to provide accurate protein structure prediction.
In 2018, DeepMind[8,9] joined and won the competition with its artificial intelligence (AI) system AlphaFold. In the 2020 competition, AlphaFold2 made another huge leap forward, predicting more than 90 % of the protein structures with experimental accuracy, leaving its competitors in the dust.[9–11]
DeepMind is a Google-owned company best known for its AI systems that can beat human players at games like chess, Go, and StarCraft. In 2017, their AI AlphaGo beat the world’s best Go player. The AI was then repurposed and learned chess without any human input.
AlphaFold2 is an AI based on deep-learning; the AI was given more than 100 000 known protein folds for training, leveraging the work of hundreds of scientists. It then uses the patterns learned from the training set to predict accurate protein structures in only a few days.
What is the impact for science and society?
DeepMind says it plans to use AlphaFold to solve the structures of proteins involved in human disease and help design drugs. Structural information can also aid the engineering of enzymes that degrade plastics or make biofuels. Since solving protein structures experimentally is expensive and time-consuming, accurate protein structure prediction could drastically speed up and lower the cost of such research.
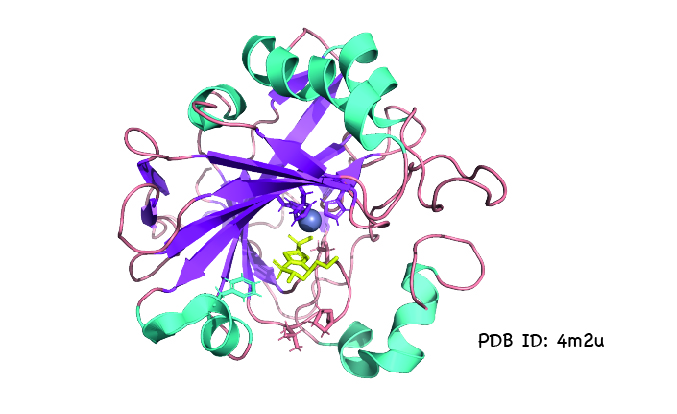
Image courtesy of Simone Heber
However, open questions remain because although machine-learning methods can provide structure predictions, they do not explain how proteins fold. If the protein tried all 10300 possible structures, it would need longer than the age of the universe to fold, yet in nature, proteins can fold in milliseconds. This is referred to as the “Levinthal paradox”, after Cyrus Levinthal who postulated it in 1969.
Furthermore, protein structures have a certain flexibility, for example when binding to another protein or a drug, so their folding depends on more than the primary sequence. This means that even with accurate structure prediction, experimental structure determination and functional investigation will still be important.
However, accurate structure prediction has the potential to speed up scientific progress and save a lot of costly, tedious experiments. Predictions can guide the design of experiments, streamlining the scientific process and allowing scientists to tackle more advanced problems faster.
References
[1] Chemistry Nobel lecture 1962, Speed read: https://www.nobelprize.org/prizes/chemistry/1962/speedread/
[2] Chemistry Nobel lecture 2002, Press release: https://www.nobelprize.org/prizes/chemistry/2002/press-release/
[3] Chemistry Nobel lecture 2017, Press release: https://www.nobelprize.org/prizes/chemistry/2017/press-release/
[4] Homepage of the protein data bank: http://www.rcsb.org/
[5] Chemistry Nobel lecture 1972, Press release: https://www.nobelprize.org/prizes/chemistry/1972/press-release/
[6] Homepage of the Folding@home computing project: https://foldingathome.org/
[7] Protein Structure Prediction Center: https://predictioncenter.org/
[8] Alphafold webpage: https://deepmind.com/research/case-studies/alphafold
[9] A Nature news article on Alphafold: https://www.nature.com/articles/d41586-020-03348-4
[10] MIT technology review: https://www.technologyreview.com/2020/11/30/1012712/deepmind-protein-folding-ai-solved-biology-science-drugs-disease/
[11] A researcher’s perspective on AlphaFold2: https://www.asbmb.org/asbmb-today/science/120520/ai-makes-huge-progress-predicting-how-proteins-fol
Resources
- Teach evolution and biochemistry with online biological databases: Tenorio G (2014) Using biological databases to teach evolution and biochemistry. Science in School 29:30–34.
- Discover how the structure of the green fluorescent protein determines its light-emission properties: Furtado S (2009) Painting life green: GFP. Science in School 12:19–23.
- Read more about protein crystallization and ESRF: Cornuéjols D (2009) Biological crystals: at the interface between physics, chemistry and biology. Science in School 11:70–76.
- Learn about bioinformatic data storage at EMBL-EBI: Stroe O (2018) Bioinformatics: the new ‘cabinet of curiosities’. Science in School 44:20–24.
- Watch a cartoon about protein structure and folding.
Institutions
Review
Treatment of diseases such as Alzheimer’s, cancer or infectious diseases depends heavily on the identification of the molecular targets for the design of new medicines. Computer technologies and artificial intelligence cut down the time and money to make it. This article brings our students to the very essence of interdisciplinary collaboration in research, as a means of fighting against diseases more efficiently.
The article would be useful in biology and chemistry teaching in order to introduce the importance of molecular 3D stuctures, in particular aminoacid sequence folding in proteins, and how artificial intelligence and bioinformatic can help us to unravel the hidden information in our cells and molecules.
Jesús López Alonso, Biology and Geology teacher, IES Gil y Carrasco-Ponferrada, Spain