Clinical trials count on more than statistics Understand article
The rush to find treatments for COVID-19 led to a badly flawed clinical trial influencing medical treatment worldwide. What went wrong?
Why do we need clinical trials?
When patients with COVID-19 began appearing in hospitals, there was no script for the doctors to follow. It was a new disease and there were no treatments known to work. Creating a new drug takes years, so the medical community had to use what was available.
Ventilators helped to relieve patients’ low oxygen levels, steroids prevented the immune system from going into overdrive, and a malaria drug called hydroxychloroquine was given in the hope that it would help clear the infection.
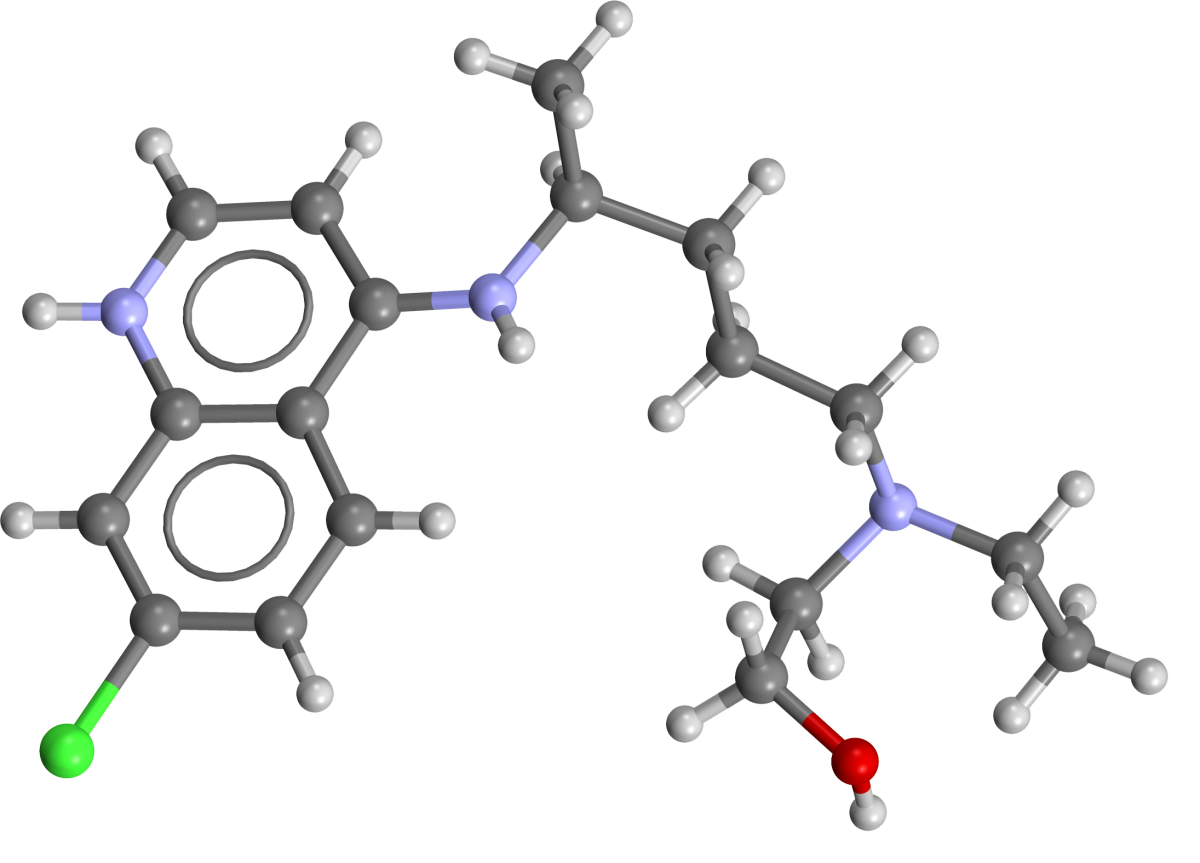
approved drug for the treatment
of malaria and certain
autoimmune conditions like lupus
and rheumatoid arthritis.
Image: Ben Mills/Wikimedia, Public Domain
With just one patient, it is impossible to say whether a new treatment made a difference or not. Perhaps that person would have gotten better on their own; perhaps the treatment caused side effects that made them worse. We cannot know without a comparison.
Any new treatment must go through a clinical trial to understand whether it is effective or not. This involves giving one group of patients the treatment and another group of similar patients a placebo (a dummy treatment that has no effect) or the usual care.
In March 2020, results from a small clinical trial of hydroxychloroquine suggested it could cure all cases of COVID-19 when combined with an antibiotic called azithromycin.[1] This led to huge public interest in the drug and health services around the world bought up stocks and treated patients with it.
Despite the attention from the media and public figures, the hydroxychloroquine trial was quickly criticised by scientists for the way that it was carried out and analysed. Larger studies over the following months found that hydroxychloroquine was not only ineffective against COVID-19 but had serious side effects.[2]
Clinical trial design
Statistics and sample size
When analysing scientific results, we first have to start with the null hypothesis, which should be our assumption before doing the study. In this case, the null hypothesis is that the treatment does not affect the patients’ recovery.
We know that some people will get better without treatment, so it is important to know the probability that we would see positive results even if the treatment had no effect. This is a commonly used statistic in science known as the p-value. A p-value of less than 0.05 means that if the null hypothesis is true, there is only a 5% probability of seeing results of this size or greater due to random chance.
This value of 0.05 is often used as a benchmark in science for results to be considered statistically significant. The hydroxychloroquine trial results met this level of statistical significance, which meant they were considered positive results.
However, a p-value of under 0.05 does not mean that the null hypothesis is wrong.
The headline figure of 100% in the trial effectiveness was based on six patients who received hydroxychloroquine and azithromycin. The sample size of a study is a very important factor in understanding the likelihood that a result is due to chance.
In any study, there will be random differences between patients. These differences will affect how they respond to the treatment and change over time. In a small group of patients, there is a greater risk that these random differences could be enough to affect the results.
Imagine doing a survey to see if women were taller than men on average. Four women and four men are selected and measured:
Women | Men | |
---|---|---|
Height (cm) | 176
178 180 182 |
172
176 174 178 |
Arithmetic
mean (cm) |
179 | 175 |
The women in the study are on average 4 cm taller than the men. This difference would be enough for a statistically significant p-value of 0.035, yet we know that women are not taller than men on average. By selecting only a small group, we have found a sample by chance that is not representative of the general population.
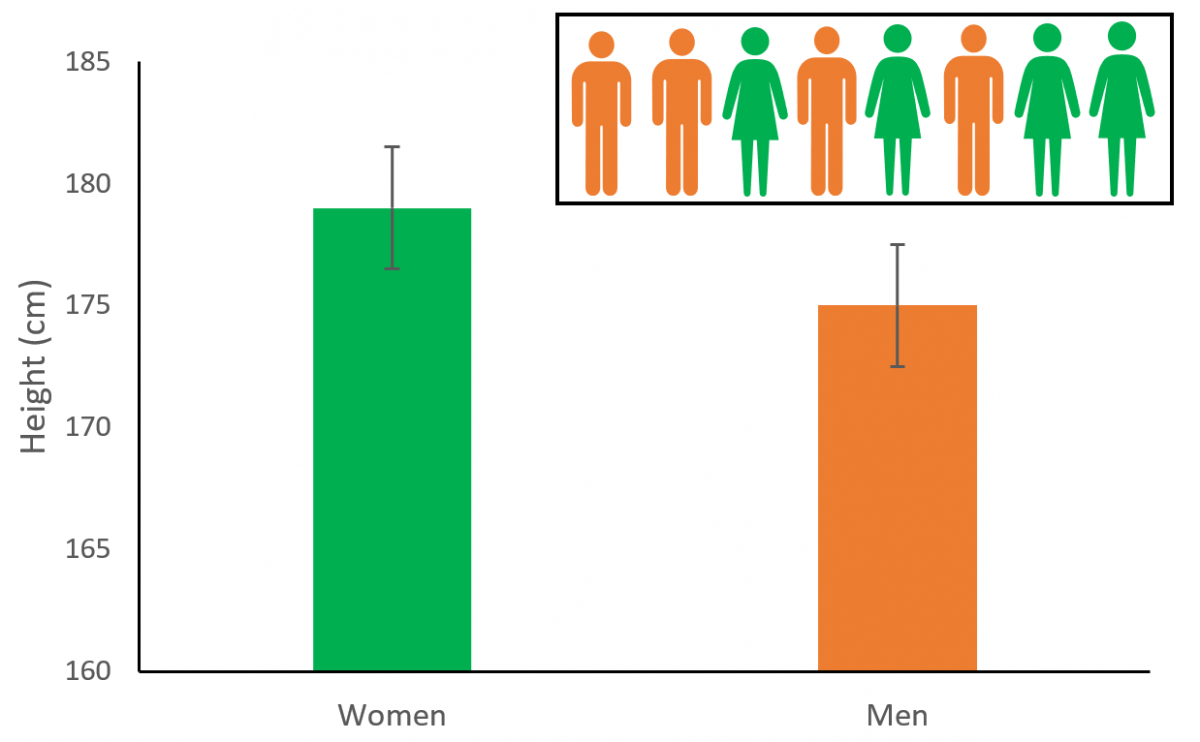
Image courtesy of Ian Le Guillou
Even with a larger group, the p-value is not enough on its own. Scientists need take into account the circumstances of the study in order to interpret a result. How many different samples were measured before getting a positive result? Were the women selected from a basketball team? Were there any differences in age between the groups? Was it decided in advance to measure only four people per group?
The answers to all of these would influence how we interpret the results. This is why clinical trials must be very carefully designed to avoid introducing bias to the results.
So what went wrong with hydroxychloroquine?
Clinical trials are highly complex studies, requiring large teams of experts to complete them successfully. However, there are a few core principles that are typically applied to ensure reliable results, including:
Key features of a well-designed clinical trial
- A control group: half of the patients receive the current standard treatment or a placebo, while the other half receives the new treatment. These groups should be as similar as possible to avoid any other effects that could influence the results.
- Randomisation: the patients should be randomly assigned to either the control group or the treatment group. This avoids any bias from either the patients or their doctors deciding who should be in which group.
- Intention-to-treat principle: the analysis should be based on the groups the patients were assigned, even if they did not complete the treatment. This helps to avoid bias if some patients drop out because they become worse while on the treatment.
- Blinding: Where possible, the patients and the doctors treating them should not know who is receiving the tested treatment versus the standard treatment or placebo. This helps to reduce the influence of their expectations (placebo effect) and experimental bias.
- Sufficient power: the study groups should be large enough to minimize apparently significant effects appearing due to random chance.
The hydroxychloroquine trial did not meet any of these measures. The control group included patients treated at different hospitals, which tested for COVID-19 differently. The patients were not randomised, with patients deciding whether or not to receive hydroxychloroquine and doctors selecting patients to also receive azithromycin. The results analysis excluded patients if they did not complete six days of treatment, including those that died or needed intensive care.
With these issues affecting the trial, the results should carry little weight even if the statistics suggest they are significant. Indeed, many scientists did raise concerns. However, political pressure and possibly desperation for an effective treatment led many to pin their hopes on hydroxychloroquine.
Impact
The knock-on effects from this trial were wide-reaching. Doctors around the world treated COVID-19 patients with a drug that provided no benefit to them and can have serious side effects, including for the eyes and heart. Health systems spent money on stocking hydroxychloroquine that could have been better spent on ventilators or personal protective equipment for staff. Furthermore, the surge in demand for this drug led to shortages for people who rely on it to treat serious illnesses like lupus or rheumatoid arthritis.
Worse still, the focus on hydroxychloroquine delayed the investigation of other treatments for COVID-19. The incorrect belief that the results of the initial badly run trial were exceptional led to hundreds of hydroxychloroquine trials being started worldwide.
Leaflet | © OpenStreetMap contributors © CARTO
Some further well-designed trials were needed to establish that hydroxychloroquine does not actually work against COVID-19; a negative result is still useful. However, so many simultaneous trials of the same drug diverted limited resources away from testing treatments that we now know are effective.[3,4]
It is impossible to quantify the damage of this debacle, but it is clear that rushing to believe in a miracle cure costs lives.
References
[1] Gautret P et al. (2020) Hydroxychloroquine and azithromycin as a treatment of COVID-19: results of an open-label non-randomized clinical trial. Int J Antimicrob Agents 56:105949. doi: 10.1016/j.ijantimicag.2020.105949
[2] The RECOVERY Collaborative Group (2020) Effect of Hydroxychloroquine in Hospitalized Patients with Covid-19. N Engl J Med 383:2030–2040. doi: 10.1056/NEJMoa2022926
[3] Ledford H (2020) Chloroquine hype is derailing the search for coronavirus treatments. Nature 580:573.
[4] Águas R (2021) Potential health and economic impacts of dexamethasone treatment for patients with COVID-19. Nat. Commun 12:915. doi: 10.1038/s41467-021-21134-2
Resources
- A detailed look at the placebo effect: Brown A (2011) Just the placebo effect? Science in School 21:52–56
- Find some classroom activities on disease spread and school mathematics: Kucharski A, Wenham C, Conlan A, Eames K (2017) Disease dynamics: understanding the spread of diseases. Science in School 40:52–56.
- An overview of Eurostat statistics that you can use with your students: https://ec.europa.eu/eurostat/help/education-corner/teachers-and-students
- Find and isolate the diseased in this game on disease spreading: https://infektionsviden.aau.dk/en/infectiondetective/
- How to evaluate a medical treatment: Garner S, Thomas R (2010) Evaluating a medical treatment. Science in School 16:54–59.